|
![]() |
At SANSA-Stack, when in search for a big data science and engineering framework, we look for one key thing: productivity. Big data science and engineering need a framework for much better productivity. Instead of viewing things bottom-up, we take a top-down view of the big data stack, and ask what kind of API we would want to maximize data-engineering productivity. We ended up with developing the SANSA-Stack, a Distributed Structured ML framework.
Read / Write RDF / OWL Library
This read / write library provides the facility to read RDF or OWL data from HDFS or a local drive supporting a wide range of formats and represent it in the native distributed data structures of the frameworks. We also provide a dedicated serialization mechanism for faster I/O. We support Jena and OWL API interfaces for processing RDF and OWL data, respectively. This particularly targets usability, as many users are already familiar with the corresponding libraries. Furthermore, this layer can compute dataset statistics/quality checks and apply functions to URIs, literals, subjects, objects.
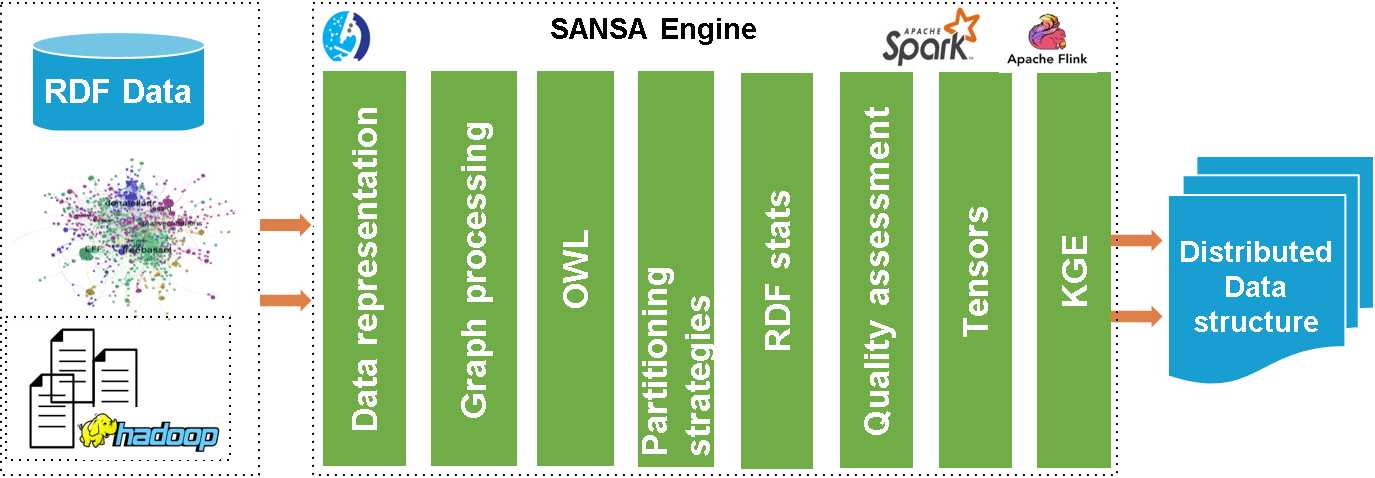
Querying Library
Querying is essential to browse, search and explore the structured information. SPARQL, the RDF query language, is very expressive and allows to extract complex relationships. SPARQL takes the description in the form of a query and returns that information in the form of a set of bindings or an RDF graph.
SANSA provides methods to perform queries directly in programs instead of writing the code corresponding to those queries (grouping, sorting, filtering etc.). It also provides a W3C standard compliant SPARQL endpoint for externally querying data that has been loaded using SANSA.
SANSA also allows to query heterogeneous and large data sources via its SANSA-DataLake component powered by a specialized Semantic Data Lake engine.
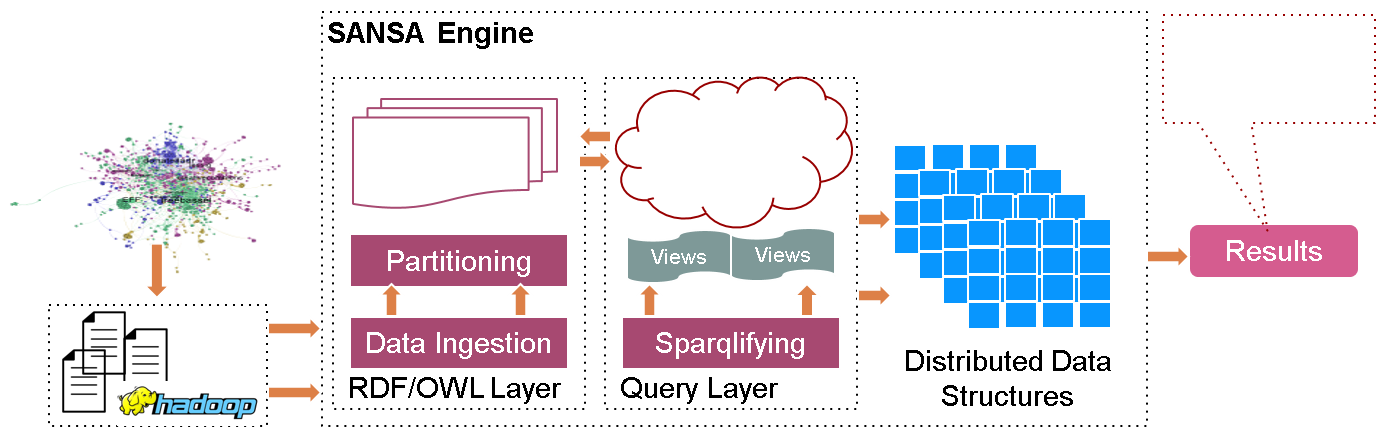
Inference Library
Both RDFS and OWL contain schema information in addition to links between different resources. This additional information and rules allows to perform reasoning on the knowledge bases in order to infer new knowledge and expanding the existing one. The core of the inference process is to continuously apply schema related rules on the input data to infer new facts. This process is helpful for deriving new knowledge and for detecting inconsistencies. SANSA provides an adaptive rule engine that can use a given set of rules and derive an efficient execution plan from those.
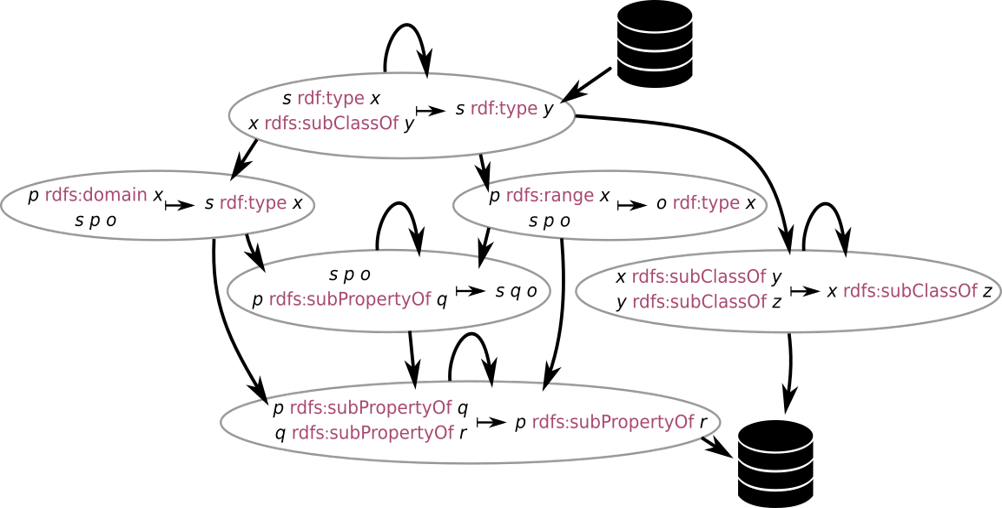
Machine Learning Library
The machine learning algorithms in this layer exploit the graph structure and semantics of the background knowledge specified using the RDF and OWL standards. In many cases, this allows obtaining either more accurate or more human-understandable results. The ML layer supports various algorithms, for example, Automatic Sparql based feature extraction through Literal2Feature, Semantic Similarity Estimation through DistSim and SimE4KG, Anomaly Detection by DistAD and construction of Downstream Pipelines by the use of DistRDF2ML. User Guide.